The data market and the various sectors using data have shown the need to go beyond the anonymization of data contained in simple tabular formats. Of course, at Octopize, our teams continue to work on improving the anonymization of tabular data, but our R&D work now allows us to manage other types of data such as:
- time series (more info in this item),
- relational databases (more information on this data) hither),
- geolocation data
Let's focus on these today...
Geolocation data
Geolocation data is a position generally defined by two variables: Its longitude and its latitude (a GPS point for example). This data can be found in a classic tabular data set (to define a person's home for example) or in the form of several pairs of latitude and longitude (to define an individual's home and workplace). In some datasets, it is a sequence of geolocation points: a trace. These points are often associated with time variables representing the moment of passage at each of these points. We then talk about timestamp traces. Such traces can be found with the Google maps history of an individual, for example.
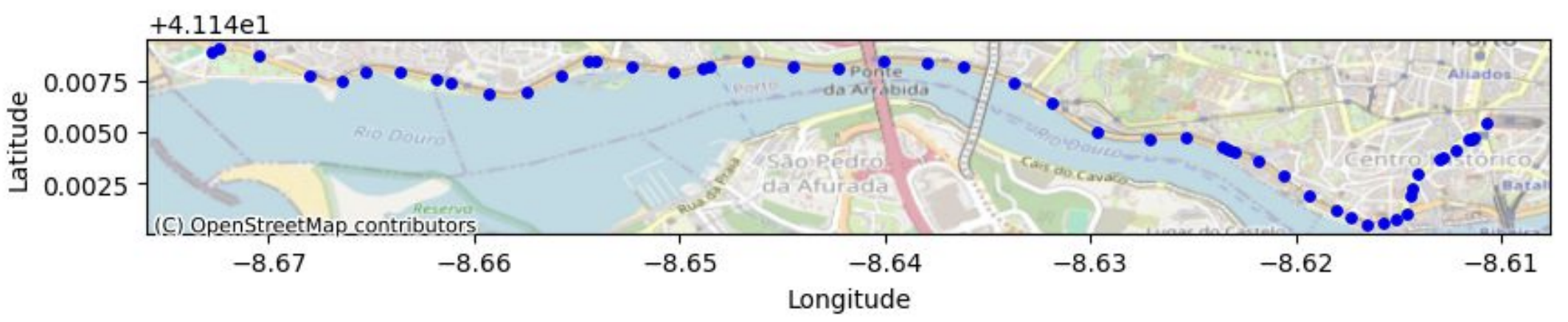

The timestamp tracks contain a very large amount of information which can be used to infer numerous sensitive details about a person such as religion (regular visits to religious buildings), health conditions (visits to specialized clinics), or any activity that he or she would like to keep private. One study reference shows that 4 points in a trace are enough to re-identify 95% of individuals in a data set because our individual movements are so unique. The LINC (CNIL Digital Innovation Laboratory) also looked into the subject and demonstrated that it was very easy to trace individuals using pseudonymized data made available by data brokers (link to the study). hither). This highlights the difficulty of anonymizing complete traces very often containing much more than 4 points...
Anonymization of geolocation data
At Octopize, we accept this challenge and work on 3 main areas:
- the anonymization of minimized data (currently available),
- Theprivacy assessment associated with a data set that has received an anonymization treatment or similar to it (currently available)
- and finally the anonymization of Complete traces (work in progress).
Minimization and anonymization
The Principle of minimization recommends collecting and maintaining only those variables that are useful for a particular use. In addition to being a key principle in the field of data protection, minimizing data makes it possible for anonymization And the retention Of usefulness required for use. For example, a community wishing to analyze travel flows does not need to use complete traces for this. Using only the departure and origin points of the trips allows it and their anonymization makes it possible to keep this data. without time limits.
Following this minimization approach, we suggest 4 representations that are compatible with the avatar method:
- Vectors : only the points of origin and destination are extracted before being anonymized. In particular, vectors make it possible to conduct analyses on public transport and carpooling practices.
- Aggregates : statistics on each trip are extracted (examples: length, average speed, number of stops, time spent in a speed range etc...). These aggregates are used for the purposes of user behavioral analysis as well as in predictive maintenance contexts.
- Snapshots : only the location of individuals at a specific moment is kept. Several successive snapshots can be generated and anonymized but a trace cannot be reconstructed from these snapshots. The use of snapshots has proven its worth in impact studies to understand the attractiveness of an event or the consequences of road works.
- Segments : the trips are divided and the identifiers of the trips and vehicles are removed. Once anonymized, it is impossible to reconstruct the trips. This type of representation is suitable for studies involving the analysis of infrastructure and traffic conditions.
Note that other representations that minimize the data and are compatible with anonymization can be considered. It is also possible to mix concepts from several representations. For example, for some use cases, it may be interesting to keep the origins and destinations of the trips as well as the aggregated statistics of these trips (mixture of vectors and aggregates).
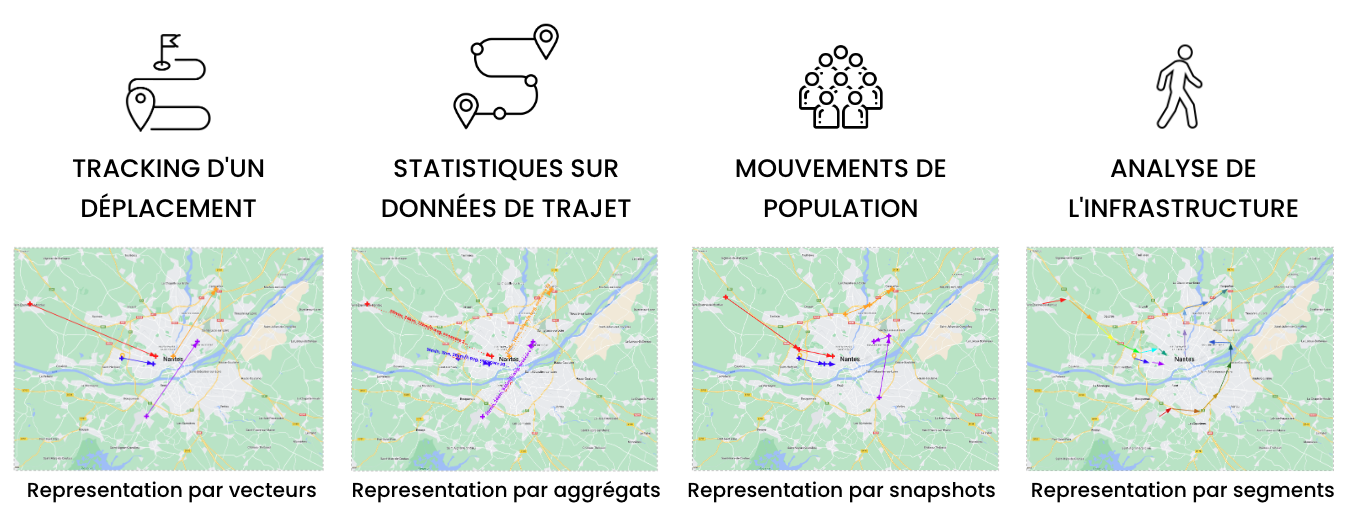
Assessment of the privacy of anonymization methods dealing with complete traces
Data-minimizing approaches can deal with a lot of use cases, but there are still contexts for which the preservation of almost raw data (in the form of complete or almost complete traces) is necessary. The state of the art (academic and industrial) on the subject is very concise and at the moment, there is no method capable of anonymizing this kind of data reliably but some companies (including Octopize) are looking into the subject.
In order to assess the result of treatments that can be applied on complete traces, we recently made available via our tool a feature to measure privacy with respect to the 3 criteria of the RGPD. This privacy assessment tool takes original data and processed data as input and generates a privacy report allowing teams working on the subject to iterate.
Anonymization of complete traces
In collaboration with Nantes Digital Sciences Laboratory (LS2N) from École Centrale Nantes in the context of a CIFRE thesis, we are working on the anonymization of complete traces. This work is beginning to bear fruit.
Below, we illustrate these statements with an example of generating synthetic traces (on the right) from a statistical modeling of the original data set (on the left). The similarity of the heat maps calculated on the original data and on the synthetic data shows the statistical similarity of the two data sets, proving the relevance of using the synthetic data set for analytical uses.
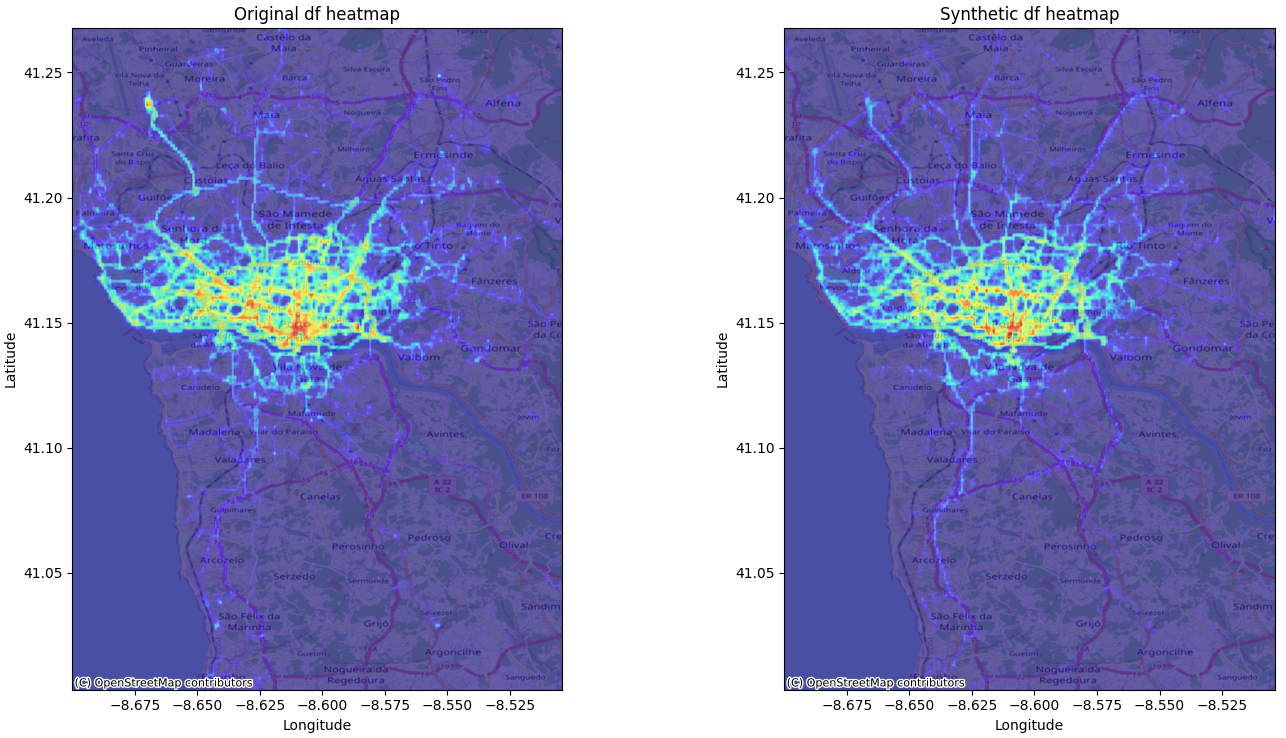
While continuing to improve the synthesis of traces from parameters, we are now also working on the anonymization of these parameters with the aim of generation of truly anonymous timestamp traces. Their anonymous nature will be evaluated using our privacy assessment brick.
This work is also part of our commitment with theTransport Innovation Agency (AIT) who accompanies us as part of the program Propulse for which we are the 2024 laureates.